Disparate systems, distributed teams, and overly complex technologies are a recipe for inefficiency in research and development (R&D) laboratories, especially in drug development. With the pressure to meet challenging deadlines and strict regulations, it is easy for working practices to become disconnected. To improve the efficiency, accuracy, and consistency of data generation, acquisition, and management, R&D organizations are looking to streamline their lab practices by investing in technologies that automate data generation, accelerate data collection speed and storage, and enhance the quality and reliability of the information produced. Lab automation presents exciting opportunities for future research, promising novel advances in machine learning and artificial intelligence (AI), liquid handling robotics, and cloud-based data workflows.
Robotics, AI, and Machine Learning
The integration of robotics and automation has revolutionized lab procedures, putting tedious manual processes in the robotic hands of automated liquid-handling systems. Lab areas that were traditionally reserved for wet lab benchwork have been reallocated to liquid handling robotics — a class of devices that range from automated pipetting systems to microplate washers — that are capable of processing large sample volumes for high throughput screening. Robotics offer preprogrammed and customizable procedures that require little supervision. Such automation saves time and effort compared to manually driven processes while improving the accuracy and reproducibility of experimental data by minimizing human error. This ultimately allows researchers to invest more time in data analysis.
Acting on a smaller scale, automation can also be used to improve data acquisition in the lab setting. For example, electronic lab notebooks are centralizing the storage of data, experimental information, and metadata relating to how an experiment has been performed (for example, environmental conditions, reagents used) while allowing new data trends to be discovered via layered analytical and visualization tools. Collecting information in this comprehensive manner would not be possible with traditional lab tools, and now individual experimental parameters can be examined where data abnormalities occur.
The implementation of automation requires effective data storage solutions that can sort, organize, and access the larger data volumes enabled by automation. Cloud-based systems are well suited to store and share large amounts of data, though the need for more efficient data analysis and data management systems has in turn translated into a surge in machine learning and AI solutions.
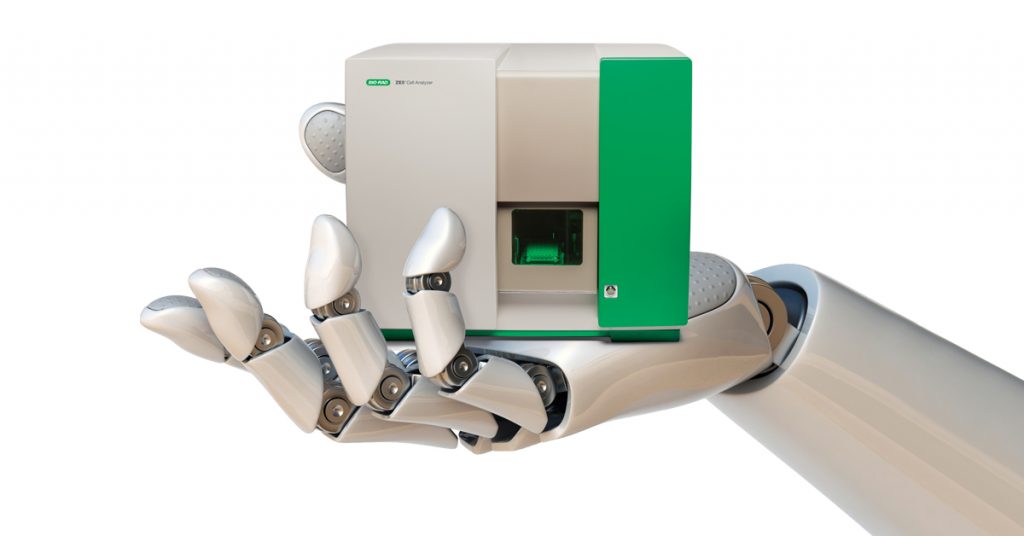
Machine learning is a branch of AI that allows researchers to more thoroughly analyze both structured and unstructured data through self-learned algorithms. In doing so, machine learning is rapidly driving hypotheses forward and directing future research. Using statistical methods, algorithms are trained to make classifications or predictions that provide key insights within large data mining projects, revolutionizing analysis and decision-making in drug discovery and development workflows.
Machine learning can also greatly support the design of experiments (DOE). For example, active learning can support the DOE by using powerful statistical approaches that explore the design space, optimizing experiments by evaluating factors that control the value of a parameter or group of parameters. Similarly, active learning can support DOE by finding the optimal algorithmic parameter settings. The use of such analytical tools is facilitated by technique familiarity and statistical experience, but also by the efficient use of automation and error-free data structuring (Freieslben et al. 2020).
Collaboration and Data Sharing via the Cloud
Technological advances in next-generation sequencing (NGS), machine learning, and cloud computing are driving faster and more cost-effective data generation workflows. The size and complexity of datasets is growing exponentially, yet with the rise of more collaborative working practices, teams are becoming increasingly geographically dispersed. Scientists and bioinformaticians are working at different sites or office locations, and many organizations are outsourcing elements of research to specialist suppliers, such as clinical research organizations. Research organizations are increasingly moving toward cloud-based systems to meet the challenges of data storage, sharing, and collaborative working.
Cloud-based platforms are a secure, robust, and cost-effective solution that enables multiple users to integrate experimental results within the same dataset and immediately access information, including historical data, via a user-friendly dashboard that can be tailored according to roles and objectives within specific user teams. A well-defined application program interface (API) provides a connection between platforms and applications, supporting cross-functional and cross-vendor collaborations, and allowing the user to control data flow within the larger ecosystem.
Find out more about why organizations are moving to the cloud in this episode of the BR.io podcast.
The Lab of the Future
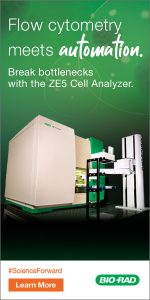
Liquid handling robotics have transformed the way experiments are conducted, and cloud-based platforms, AI, and machine learning have now made it significantly easier to access, share and analyze data. Enabled and empowered by data workflow automation, research teams find themselves in a truly win-win situation: datasets are easier to integrate and access within the system and they are of greater value because unlocking the data’s true potential is easier.
Scientists at the start of their careers are increasingly motivated by the prospect of automation and the freedom to explore their data using tools that are not traditionally found within a lab environment. Realizing the potential of automation requires that researchers possess skills in both information technology and lab-based research. When it comes to lab evolution, great strides have been made in recent years and organizations that make intentional efforts to advance automation technology and provide technological support for their research staff are sure to bring scientists even closer to an almost fully automated “lab of the future.”
Learn more about the lab of the future in this episode of the BR.io podcast.
References
Freiesleben J, Keim J, and Grutsch M (2020). Machine learning and Design of Experiments: Alternative approaches or complementary methodologies for quality improvement? Qual Reliab Eng Int 36, 1837–1848.