Manufacturing and high-throughput screening may seem to have very little in common, but they are related at a fundamental level. Both seek to transform a raw material into a finished product, and both struggle with constraints, or bottlenecks, that reduce efficiency. How can we apply the revolutionary 20th century management principles of continuous improvement to 21st century flow cytometry?
Dr. Eliyahu M. Goldratt was a business management guru and the originator of the Optimized Production Technique and Theory of Constraints — topics explored in his book The Goal, published in 1984. In The Goal, the main character, Alex Rogo, manages a production plant with an uncertain future. The plant is failing, and the protagonist has only 90 days to turn it around. Fortunately, a chance meeting with a former colleague helps him break out of conventional ways of thinking to see what needs to be done. In this way, the book describes the role of bottlenecks, or constraints, in manufacturing processes and reveals how identifying them makes it possible to reduce their impact. The plot revolves around finding and addressing the current limiting constraint, followed by finding the next one, and so on.
There are common principles that can be applied to manufacturing and flow cytometry. If we think of a flow cytometry screening campaign in the same way as a manufacturing process, we can begin to explore and pinpoint the system’s constraints. The goal is to identify a subset of samples with promising characteristics. To do this, samples are processed and turned into data. These data are then analyzed and converted into results.
There are many variables that interact to determine where the constraints in the system lie, but we can make some general assumptions. First, let us assume that the “raw materials”, in this case, samples, are in excess — an assumption admittedly far from a given. Now, let’s assume that in keeping with most screening assays, the data complexity is relatively low, with no more than ten measured parameters from each sample. Modern data analysis packages can process hundreds of data files of this level of complexity in seconds. However, the fastest flow cytometer would require minutes to acquire the data from only 100 samples. Clearly, the flow cytometer is the bottleneck in this process.
The obvious solution to lift this constraint is to invest in the instrument that can process samples at the fastest rate and increase capacity by purchasing multiple units until the constraint is removed. But what does “fastest” mean in this context? One definition may be to prioritize moving between samples quickly, perhaps at the expense of the amount of data generated from each sample. Alternatively, a much larger total volume of data could be collected in a shorter time but from fewer samples. Of course, ideally, we want a large volume of data from a large number of samples. Choosing an instrument that is low maintenance and suffers from as little downtime as possible is also important. Impressive claims of speed in instrument specifications are meaningless if the instrument is out of action due to a sample line blockage before the run is completed. This is a problem that is all too common in some screening instruments.
Obviously, we can’t just keep buying instruments and expect productivity to increase linearly. Sooner or later, we start to encounter the second bottleneck: workforce. In the case of a single instrument, one user can reasonably be expected to prepare and load the samples, run the instrument, export the data files, and analyze the data. But even that could be a stretch in some cases, and running two or more instruments at capacity for several days is simply not realistic. Of course, this problem could be addressed by recruiting more staff, but this is far more challenging than buying additional equipment; even the most dedicated employees need rest. On the other hand, an automated system can work through the night, never tires of tedious, repetitive actions, and can even deliver improved reproducibility.
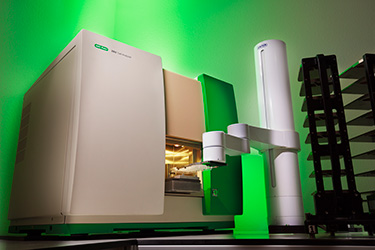
The speed and automation capabilities of the ZE5 Cell Analyzer may be the solution to your workflow bottleneck.
Again, the choice of cytometer is critical here as it must be automation-ready. Consider steps typically performed manually. These steps could include agitating the samples, keeping the samples cool, cleaning and flushing the sample line between plates, and cleaning on shutdown. Automation is unlikely a viable option if these operations are critical and can’t be performed by the cytometer’s software alone.
At a more fundamental level, the cytometer must have an application programming interface, or API, without which automation is practically impossible. However, this is a minimum requirement, and not all APIs allow the same level of functionality. An API works like an interpreter, allowing communication between two programs — in this case, the software running the instrument and the scheduling software. The commands that the API has access to are referred to as the API library, but an inadequate API library can severely limit functionality. Suppose the API cannot perform functions such as automatic fault recovery (for example, in the case of a blockage) or cannot perform maintenance functions like cleaning the instrument. In this case, it’s likely to necessitate regular user intervention or even supervision, negating the whole point of automation. An instrument’s API quality is challenging to assess, even for the most experienced scientist. For a clear and unbiased opinion about which instruments work best with automation, your chosen integrator (the person or company bringing together the components of your automation system) will be happy to advise you. Just remember to speak with your integrator before you make your instrument purchase.
The ZE5 Cell Analyzer is the first screening flow cytometer that comprehensively addresses both the first and second constraints. This analyzer can collect individual samples at an industry-leading speed of 100,000 events/second and moves between samples faster than almost any other cytometer. Its reliable fluidics with reversible pump and unclog features minimize downtime, meaning that the ZE5 Cell Analyzer is not just fast on paper but is functionally fast. Its API has been designed to provide maximum flexibility with features that ensure that user intervention is kept to a minimum, with automated fault detection and recovery and automated cleaning on shutdown. Moreover, the previous description of a typical screening assay of high sample numbers and low complexity is not universal; screening assays can now be complex. The ZE5 Cell Analyzer can perform up to 30 parameter experiments as quickly as it can ten. Thanks to the ZE5 Cell Analyzer, nearly any flow cytometry application can be run at the speed of a screening instrument.
This is not the end; in the law of bottlenecks, clearing one only reveals the next. It is increasingly recognized that as constraints begin to be overcome and the data collection rate increases, we need to develop new strategies to analyze and interpret those data. Machine learning techniques are increasingly recognized as useful tools in this context. Dimensional reduction using tools such as t-distributed stochastic neighbor embedding (t-SNE) and uniform manifold approximation and projection (UMAP) enables researchers to visualize high-dimensional data in two- or three-dimensional plots to recognize patterns and convey biological insights. Automated methods of cell population identification can be used to measure established cell populations and to discover novel subsets. Machine learning can also be used for sample classification to predict clinical or biological outcomes, such as predicting who will respond to a particular treatment or identifying healthy versus disease states. As the trickle in flow cytometry data begins to transform into a flood, AI and machine learning look set to provide useful tools to realize our newfound data wealth.
If you have ever worked in a cytometry lab, you will be familiar with rooms containing multiple instruments and users standing around them waiting to load the next tube or plate. Next time, ask yourself why they look like that and if a single instrument could handle the same workload and do it unsupervised. It may be quite satisfying as a user to see your data displayed in real time or to pull the answer out of the data after a day of solid analysis. But just like Alex Rogo, perhaps it’s time for us all to break out of conventional ways of thinking and embrace new production methods.
Learn more about how the ZE5 Cell Analyzer can break bottlenecks in your workflow.
Further Reading
Cowan WM et al. (1997). Molecular and Cellular Approaches to Neural Development (New York: Oxford University Press).