It’s hard to imagine the Human Genome Project was completed nearly two decades ago, in 2003. The primary vision of this project was to produce a human reference genome, a representative example of the set of genes in one idealized individual human. Within the genome, particularly in areas where there is very little variation among people, the reference genome has proven to be an invaluable tool. This genome has significantly progressed our understanding across many facets of human genomics research and discovery. Since the first human genome was completed, there have been several follow-on projects to refine and finish the genome. However, the currently available reference genomes do not represent the genetic diversity found across different human populations. As such, any downstream products (drug therapies, personalized medicine, etc.) derived from the human genome will not accurately represent the vast majority of the human population.
The Backstory
The Human Genome Project used a technique called “mosaic,” which involved sequencing a small number of individuals and then assembling them to create a complete sequence for each chromosome. Although the human reference genome was created using this mosaic approach, so it doesn’t represent any one individual, its reliance on the DNA of only a few people limits its utility. It is reported that over 70% of its sequences come from a single donor. Since almost all genetic sequencing experiments and discoveries rely entirely on the human reference genome, there is a pressing need to improve the reference to better capture the diversity found in different human populations.
The Problem of Sampling Bias
In the last decade, through thousands of genome-wide association studies (GWAS), scientists have made significant improvements to our understanding of complex chronic diseases, such as Alzheimer’s and type 2 diabetes, from a genetics perspective. These GWAS studies search across the genomes of thousands of people for known genetic variants or single-nucleotide polymorphisms (SNPs) to discover which variants are associated with a specific disease, condition, or state. These studies may have found, for example, variant genes responsible for encoding drug-metabolizing enzymes or drug targets, which can then be used to predict human drug responses or a drug’s toxicity.
Using SNPs to study the genetics of drug response also helps in the discovery of personalized medicine options, the most appropriate drug for a given individual. These same SNPs can also be used to predict dosing and potential toxicity, as SNPs can affect the absorbance and clearance of therapeutic agents. Finally, SNPs can be used as potential targets for discovery and development of novel drug therapies. The problem, however, is that 96% of the subjects in most of these GWAS studies are people of European descent (Need and Goldstein 2009). Unfortunately, if nothing changes, this type of sampling bias is likely to persist in future research slowing down the discovery of novel, effective and safe therapeutics and appropriate personalized treatments.
Addressing the Gap
As next-generation sequencing (NGS) itself has become more ubiquitous globally, more scientists have gained expertise and training in wet-lab techniques. However, the bioinformatics challenges seem to persist for a variety of reasons. Difficulty obtaining the requisite hardware and software is certainly one aspect of this challenge. Additionally, the data needed to generate the background (of known genetic variants) to do these comparisons often do not exist. Initiatives such as the 1,000 Genomes Project are underway, which is an excellent first step to providing a broader reference resource for researchers worldwide. This project aims to catalog all genetic variants occurring in more than 1% of various populations throughout the world, including diverse populations from Africa, Europe, the Far East, and South Asia. Bolstering genomic studies globally will also require fostering collaboration between countries and industry and enable the transfer of both funding and technology.
Researchers have assessed the ability to generalize the data from GWAS and other functional studies’ discoveries between different populations. Their data suggest that findings from one population may not be easily translated to other populations. Researchers worldwide must expand the way they tackle human genomics and take care to include racial and ethnic minorities. If we do not change this narrow approach, we will find ourselves with a very biased understanding of which variants are important, and the great potential of genomic medicine will continue to benefit only a few.
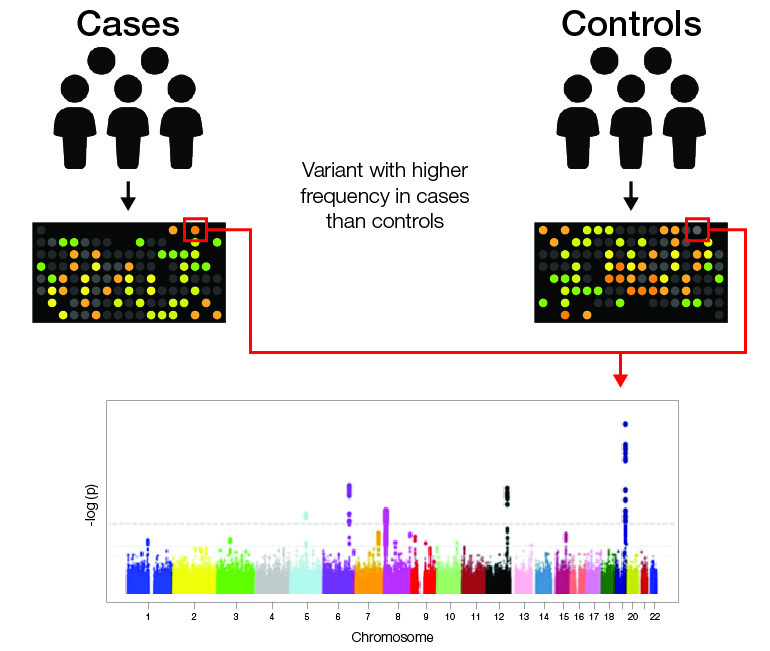
Diagram to show the identification of alternative variants in cases and controls using GWAS. Results are subject to statistical analyses to assign a p-value to each variant. https://www.ebi.ac.uk/training/online/course/gwas-catalog-exploring-snp-trait-associations-2019/what-gwas-catalog/what-are-genome-wide
References
Need AC and Goldstein DB (2009). Next generation disparities in human genomics: concerns and remedies. Trends Genet 25, 489–494.